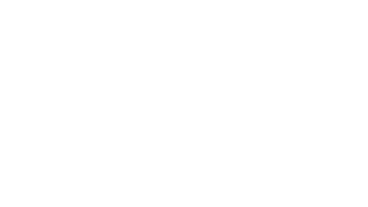
- Start date
- Duration
- Format
- Language
- 14 May 2025
- 9 days
- Class
- Italian
Per ampliare la propria visione attraverso soluzioni ad ampio spettro in grado di generare valore.
Making predictions a key managerial activity which enables organizations to focus the attention of management on the future with a dual goal. On one hand, predictions are necessary to try to understand the dynamics of the company and the context in which company leaders do their work (for example scenario analysis prior to decision making); on the other, predictions serve to assign objectives so they can focus their attention and their behaviors (a typical example here is target setting).
Proof of the essential nature of prediction lies in the fact that there are numerous managerial processes that hinge on the “predictive moment”: strategic planning, budgeting, forecasting, and operational planning are just a few small examples that confirm this central role. But despite the importance of this moment, many studies underscore dissatisfaction among the people who actively participate in planning and/or those who utilize relative processes. There are various reasons for this dissatisfaction: the low quality of predictions, the major effort expended on this activity, the disconnect between various phases in the prediction process. This dissatisfaction seems to stem from budgeting and forecasting in particular. But why? The motivations seem to relate less to the usefulness of the processes and more to the practices with which most companies have activated these processes over time, practices which may have been considered adequate up till quite recently. But in the past few years their limitations have emerged.
The first has to do with the approach to predictions: specifically, the processes of budgeting and forecasting nearly always follow a continuous line with the past. In fact, the past is our starting point when we make predictions, and based on the past, we make adjustments to expected results for the coming period or year. The second limitation has to do with the object of predictions. In the vast majority of cases, predictions relate to expected results, but underlying these there may be multiple drivers and dynamics that differ widely.
Clearly, with business cycles becoming shorter in duration and constantly changing in terms of performance drivers, this dual assumption is proving wholly inadequate as far as the quality of predictions, all the more so in times of profound discontinuity like we are seeing today. With the pandemic we are living through and all the implications (both contingent and structural) that we will have to contend with in upcoming months and years, past dynamics give us few useful indications for predicting the future. So we need to totally rethink all the typical predictive processes in companies, starting with budgeting and forecasting.
Three elements of innovation are necessary to upgrade these processes to match the characteristics of the current context. The first relates to the need both to understand the business determinants underpinning the process of generating results, and to separate context determinants (which company managers have little control over) from company determinants (which instead fall into the sphere of managerial influence). The second is to anchor efforts to predict these determinants in an attempt to understand how they will move in future months and years. The third is to comprehend how changes in these determinants can impact the process of generating future results. In other words, what we actually have to predict are performance drivers. What’s more, we can make performance scenarios more reliable if we can figure out how the evolution of these drivers could potentially condition company results.
In the short term, this different prediction approach may be useful to try to distinguish what portion of the performance dynamics we can attribute to context variables (specifically the pandemic and the consequent economic implications), and instead what portion we can ascribe to the strategic and operational choices that companies are making right now. In the medium term, this same approach would enable companies to improve their prediction capabilities, keeping pace with an increasingly dynamic and changeable competitive context.
Making predictions based on business drivers can also facilitate the diagnostic capacity of the company when differentials emerge between expected results and actual results. By attributing these results to their underlying determinants, we can fully understand which drivers (contextual or internal) cause performance gaps. At this point, companies can activate formal circuits for managerial learning that would make their prediction efforts more useful and effective.
In fact, this need has been emerging for some time in the business world, but only recently more evolved companies have acknowledged and responded it. Technology in this context serves as a powerful facilitator because companies can conduct far more extensive research and analysis on the relationships between performance and determinants than in the past.
In any case, we are living in a period of time that may represent an opportunity to further accelerate this path and lead many companies to rethink prediction cycles to augment their use and effectiveness. This is one of the managerial challenges we may be forced to face in these difficult times.