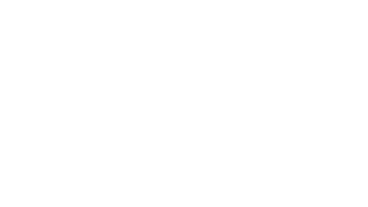
- Start date
- Duration
- Format
- Language
- 16 Sep 2025
- 40 hours
- Online
- Italian
Fornire gli strumenti per (ri)disegnare la roadmap di adozione e sviluppo dell’AI in azienda bilanciando strategia, elementi tecnologici, organizzativi e di contesto.
No one has any doubt by now about the importance of data to support decisions. Because of the speed, the globality and the transversality that typify decision-making activities today, systems are essential that can transform data into utilizable information in an instantaneous and integrated way.
At the same time, technology has fostered the development of increasingly sophisticated and fascinating methods for data analysis. As often happens, the combination of need and opportunity produced a solution: machine learning.
The idea here is that a model can be suggested by the data and shaped by their variability and variety. In the world of chess, for instance, greats like Kasparov, Fischer and Carlsen learned to play from books, and by studying the games played by the champions who came before them, eventually becoming good enough to beat them. Likewise, a machine learning (ML) algorithm (or deep learning even more), trained in the basics of mathematical/statistical analysis, can learn and improve with every analysis it runs.
So has ML killed classical statistics as we’ve known it till today, made up of more or less rigid models we try to bend our data to fit? If, as always, we try to base our conclusions on the data, the answer would be a resounding no.
Looking at several studies, the most recent conducted in late 2020 by Kaggle (the biggest data science community in the world), we can see that classic models are still adopted far more often to turn a datum into information to support decision-making. The findings of Kaggle’s research may come as a surprise for some: 76% of the ten thousand plus professionals who participated in the study said that the algorithms they use regularly in their work are linear regression and logistic, followed by 65% who utilize decision trees and random forest. Only 40% apply machine learning oriented techniques (neural networks, deep learning, etc.).
The question (at least for many young data scientists) is: if a Formula 1 racecar is an option, why are there still so many pickup trucks on the road?
We can find the first answer in the ambiguous content of the question itself. It’s a mistake to consider classic models as utility vehicles with respect to the high-performance car that is machine learning. Instead it makes much more sense to compare the different approaches to data analysis to different cars – a sports car but a four-by-four too, a convertible but also a van, an automatic but with a stick shift as well. I have to choose the right car to suit my needs: if I’m taking the family on vacation, with the two-seater sports car I’ll get there quicker, but where do I put the rest of the family and all the luggage? Likewise, in some situations machine learning methodology will be the most appropriate; others call for a classic approach, because the premises and the objectives are different.
Now let’s find out the main differences between the two. We won’t delve into too much detail about individual methodologies, but we’ll try to see why statistics is not dead - quite the contrary. It’s alive and well and practically irreplaceable in managerial practice.
The first fundamental difference is the quantity of available data. As we can easily imagine, machine learning techniques require vast amounts of data to learn in a deductive way, and we don’t always have such a huge database on hand. When we want to verify an existing model inductively using a traditional approach, we need less data to ascertain the validity of that model with respect to the data. In other words, researchers formulate certain hypotheses on the relationships among the variables at play, either starting from current economic and managerial theories, or based on experience in the field. Data are utilized only to confirm or controvert the significance of these relationships, and not to build new knowledge. For this reason, we can say that in a certain sense classical models are less ‘voracious’ in terms of sample sizes.
The second major difference is the objective of the analysis. If the aim is predictive performance, that is, the model’s ability to avoid mistakes (or to make as few as possible), then understanding the model is less important. From this perspective, models based on machine learning techniques are certainly highly recommended because they are performance-oriented, but hard to understand for the end user (at least the more complex techniques). If on the other hand the point is to get a grasp of a phenomenon, to read the model and its implications, and to interpret the impacts of individual variables, traditional models are the way to go because they are structured a priori in form and estimated by means of data in substance. Here we need to underscore that modern analytical tools focus more on the interpretative aspect of machine learning models.
There are also differences in the time horizons when decisions are made, and differences with respect to the weight of each decision. Let’s say for example we’re hunting for suspicious transactions. If we need to analyze thousands of credit card purchases or financial markets in real time to find what we’re looking for, we have masses of data on hand, and of course we’re interested in performance, that is, accurately identifying these operations. So here machine learning models are ideal for our purpose. But if instead we want to grasp the logic behind these shady deals, what characteristics (variables) to monitor for prevention or intervention with rules and regulations, we have to understand the model, so traditional models or the more basic and interpretable machine learning models are the best fit (random forest).
Essentially, simplifying somewhat, the more sophisticated algorithms from machine learning are very useful for analysis operational processes. On the other hand, classical models are often utilized to understand phenomena (or when the amount of data leaves on hand us with no other option).
Added to this is the fact that classic statistics is fundamental in the exploratory phase and for data preparation, which is an essential step for applying even the most complex models. (To cook gourmet food, we need to prep and taste the ingredients first. Or to use a common catchphrase among analysts, “garbage in, garbage out” is a rule that applies to machine learning too.) So as we can see, machine learning is an addition to – not a substitute for - statistics in the toolkit that data and business analysts use to accomplish their true task: to extract from the data everything possible to support decisions, in a world that is increasingly complex on one hand, and data rich on the other.