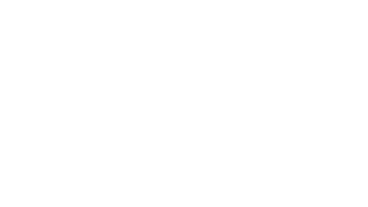
- Start Date
- Duration
- Format
- Language
- 14 Apr 2025
- 4,5 days
- Class
- Italian
Gestire in modo integrato e coerente una rete di vendita potenziando la capacità di prendere decisioni efficaci e sviluppando una visione strategica del sales management.
With public construction projects, numerous factors come into play, impacting a contractor’s chances of winning a bid. What exactly are these factors? And how do they influence a bidder’s chances of success? A recent study gives us some answers…
At an international level, the construction industry is not only vast, fragmented, and competitive – it offers relatively low profit margins. With public construction projects, when the contractors who place the lowest bid are awarded the project, a number of different factors come into play, influencing the outcome of the process. What exactly are these factors? And to what extent do they influence a contractor’s chances of success? A recent study, the basis for a scientific paper, attempts to find the answers to these questions.
With public works, except in certain emergency situations, contractors can win projects only by participating in a competitive bidding process. Since turnover among contractors is high, but the profit margins are generally quite slim, contracting is very risky business. When invited to participate in tenders, contractors face difficult decisions, firstly whether or not to tender a bid at all (i.e. bid/no-bid), and secondly which markup to use in their bid prices.
Utilizing a combination of tender data and bidder data as independent variables, we were able to build empirical models that serve to predict the outcome of tenders, determine the chances of success for bidders, and rank them accordingly.
The data set we collected for our study pertains to tenders in different countries for various types of projects in a timeframe from 2013 to 2019. Specifically:
Unlike previous studies, our data did not come from interviews with managers; instead, our work was based on empirical data on tenders and individual bidders.
By running a series of statistical analyses (including bivariate and multivariate regressions, and machine learning applications (LASSO)), we develop an econometric model that incorporates new variables (which are not addressed in extant literature), enabling us to predict the winners of the tenders in question.
In this case, in contrast with traditional research that focused on identifying a relationship between a single independent variable and the level of competitiveness, we built a predictive model based on correlations between the level of competitiveness and several independent variables. The aim of our work was to pinpoint which factors influence the chances of a bidder winning a contract, and the degree of this influence. We specifically looked at tenders for public construction projects which were awarded to the lowest bidders.
The statistical analysis for our research was particularly complicated due to certain limitations, chief among them information access. In fact, the data pertaining to any given contract/bidder are publicly available only when both the arranger of the tender and the bidder are public companies. Another limitation we had to contend with was inherent to the construction industry, where every contract is different, there are only a few bidders for each project, and for the most part the bidders are different for any given tender. Lastly, the effects of independent variables can only be verified on bid prices, not on cost estimates or profits because it is not possible to break down bids into these constituent elements.
Based on our data analysis, we developed three econometric models that incorporate five independent variables of the bidders:
Generally speaking, our findings show that the biggest impact on the chances of winning tenders come precisely from the five factors above. Specifically, current work load in other projects and the bidder’s age reduce the chances of a successful bid, while the bidder’s experience in the tender country, project-specific experience and the level of internationalization have a positive effect. The relative importance of these variables changes from model to model. What’s more, choosing which model to use depends on two conditions: whether most of the bidders are international or local, and whether bidder data are available to calculate the independent variables.
Beyond the academic contribution of our study, our findings have useful practical implications for contractors, project owners and consultants.
To predict the outcomes of tenders in a more thorough and accurate way, future research could incorporate independent variables such as the bidder’s prior experience with the tender client, the bidder’s participation in pre-tender meetings or site visits, and the experience of a specialized bidding team (in the bidding company). In addition, subsequent studies should continue not only to build econometric models with combinations of tender data and bidder data serving as variables, but also to combine these econometric models with the homogeneous and heterogeneous bidding models from previous research.
Last of all, a future focus should also be analyzing the effects of tender data and bidder data on cost estimates and profits, taken separately (beyond the total of these two figures, i.e. the bid price). The aim here would be to ascertain how these two data sets impact the components of bid prices.