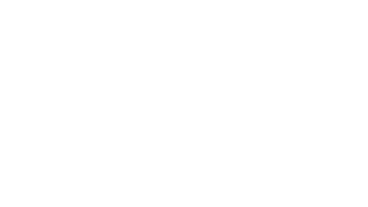
- Start date
- Duration
- Format
- Language
- 16 Jun 2025
- 9 days
- Class
- Italian
Affrontare le sfide attuali della funzione HR a 360 gradi, grazie a strumenti metodologici per attrarre, scegliere e trattenere in azienda i migliori talenti.
The Covid-19 emergency is shining a light on a phenomenon that we were already observing before the crisis, one that Gianluca Salviotti unequivocally underscores in his recent article published on this site It is this: digitalization alone does not equate to transformation; this is especially true with the ‘emergency’ digitalization we’re seeing right now. Instead what we need is a strategic vision, organizational intervention on processes, people, and operating mechanisms, and on IT structures and processes. Generally speaking, even pre-Covid, much discussion has centered on the fact that investments and digital projects would not generate tangible, long-lasting corporate results, unless there was a systemic vision that took into account many of those perennial elements, the basics of corporate management.
This means on one hand, solid business and technology strategy, encompassing process reengineering/optimization as well as change management; on the other, well-structured investment/financing plans. For many earlier generation technologies (such as the dissemination of ERP or CRM systems, or Business Intelligence/Data Analytics), studies and business experience already clearly demonstrated the need to implement technology with a clear strategic, organizational and technological vision.
In line with these observations, prestigious international journals such as the Harvard Business Review, the MIT Sloan Management Review and the MIT Technology Review have recently published articles that are critical of the digital results that companies actually attain, or cautious of many new technologies (for example the impacts of AI on ethics, or of advanced robotics on employment, or of fixed and mobile sensors on privacy).
Even closer to home, if we look at the digital results achieved by a number of Italian companies, the same doubt comes to mind. In this stage, during this rush toward digitalization, which today is code red, we may be forgetting that the traditional tenets of business management (and IT management) still count!
The innovation model is made up of business units (specialized and non), inter-functional committees or teams, different roles and accountability (with the most CXO acronyms imaginable!), innovation management processes (whether internal or open innovation, structural or agile, ISO 56000 compliant), competencies, budgets, metrics and ad hoc KPIs. And all this carries substantial weight in generating real, long-lasting results in digital transformation too.
There’s no doubt that the tempo of the past few years has been accelerating. And there are some new or revamped methods can certainly be useful facilitators (take for example design thinking, service design, agile project management, co-creation, test & learn applications, etc.). But these methods, combined with new technologies, have yet to guarantee across-the-board success in digital transformation in many sectors of application. And this is not only due to the problem of lack of skills or compliance. How many projects and digital experiences do we hear about at conferences or seminars, only to later discover that they’re nowhere to be found in our company? They’re either stuck in a pilot program or test phase, or lying dormant in some internal IT system, or on slides that get far too much media exposure.
The scalability of digital projects is certainly one of the biggest criticalities that companies face. They need to acquire the right skills to tackle scaling, use business cases to weigh economic viability, and prototype a system for customer service and digital experience, not just digital technologies as such. Even when we look to examples of innovation from the big players on the web for inspiration, we get a reality check. How much of that experience is actually replicable in traditional medium-sized companies that are in the process of going digital (i.e. non-digital natives)? If we take their organizational – and technological - models for managing and leveraging their digital-native data, to what extent can these models really inform digital enterprise or data-driven organization models used by Italian firms? And finally, we can’t help but note that all the recent research on digitalization still paints a picture of low-to-average adoption of new digital technologies in this country. What’s more, all managers at every level, when asked about the relevance and possibility of adopting these technologies, pointed to a future possibility three to five years from now.
We can find myriad insightful examples (in the context of data analytics, digital multi-channel, IoT, virtual and augmented reality, AI, etc) that suggest digital change in a “wise, routine and sustainable way,” more often incremental than disruptive, while reconsidering the basic principles of business management. Here are a few. Recent articles in the Harvard Business Review evidence how the dissemination of various analytics and the construction of data-driven organizations don’t depend on new technologies to source, or new data to analyze or new skills to acquire. Instead today as in the past, the challenge still lies in clearly defining the business units, the processes and the roles among the different data analysis experts (and not only data scientists) and the company decision makers who have to utilize the outcomes of data analysis.
Once again two evergreen elements of data analysis emerge:
Among non-digital native firms, we also find that once AI-based projects have been tested, they often languish as nothing more than niche applications, separate and apart, not integrated with other company systems. As a result these initiatives never morph into concrete products or services that have any market impact. Today we see that AI algorithms also pose problems on an ethical level, because they are created by programmers who can embed distortions both when building and teaching algorithms, and in how they learn (deriving from vastly diverse principles, values, convictions, priorities and susceptibilities).
What’s more, AI has to factor in a number of exceptions that it’s not yet capable of handling, with the examples that they’re given to train and inform machine learning algorithms (whether these consist of time series on sales, records of contact center requests, image or scene recognition). In business management, AI must take into account human experience, corporate accountability of a given decision, and a number of sensitivities in decision-making that are not easy to code into the software.
In different contexts, such as multi-channel and e-commerce, traditional companies (not pure onlines) still have a very hard time measuring business performance; they tend to rely on mainly technical indices and metrics. This while the debate continues on how to bring the online shopping experience into the physical world. What’s more, many marketing and digital managers are realizing how difficult it is to scale up to the “top seven” mobile apps that each individual uses most on their cell phones. Numerous apps still count a very small number of downloads and high cancellations, low ratings and negative reviews, damaging the company’s reputation to no small degree, all of which needs to be calculated.
Last we come to smart stores, or highly digitalized physical stores. Smart stores implement a wide range of digital technologies, and cover several different sectors (first and foremost grocery and fashion). But their success still depends a great deal on certain decisions that we could call ‘classic’: the ability to measure net results, decisions on store location and ideal size, a knack for finding the right assortment mix for target users. And then there are decisions about expanding services (for instance, supermarkets offering dine-in food, pick-up points, drive-thrus and so forth), the ability to listen to consumers and gauge their perceptions of the new in-store technologies. And last of all, the capacity to scale the format, because a few smart stores in a retail chain won’t impact the customer experience or the operational efficiency of the retailer, much less the garner interest of branded products to collaborate to support growth.