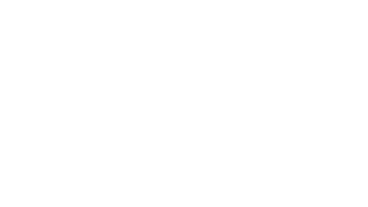
- Start date
- Duration
- Format
- Language
- 5 May 2025
- 9 days
- Class
- Italian
Affrontare le sfide attuali della funzione HR a 360 gradi, grazie a strumenti metodologici per attrarre, scegliere e trattenere in azienda i migliori talenti.
Business managers should focus on developing all the competencies they need – organizational as well as technical
Artificial Intelligence (AI) is the branch of information technology that deals with studying and creating intelligent systems that can interact with the surrounding environment and perform a series of specialized tasks. We’ve known the basics of AI for decades now, but only in the last few years have we begun to fully realize the impact on business that derives from the huge masses of available data and the incredible upsurge in computing power.
AI was traditionally based on systems that were structured around fixed rules (a given input will always give to the same output). But today, Machine Leaning (ML) makes it possible to develop systems that actually learn and complete tasks based on available data. Especially promising is the field of Deep Learning, where artificial neural networks can interconnect various layers of analysis, extending AI’s range of action to images, sounds, and texts. Beyond working on data in a strict sense, today’s AI technologies also encompass the study of natural language (Natural Language Processing or NLP), and intelligent image recognition (Intelligent Vision)
Figure 1 AI Capability Framework
Thanks to their ability to turn unstructured data into ordered, relevant information, AI technologies can help companies in many different fields accomplish any number of tasks: making increasingly sophisticated predictions (for example, on fluctuations in demand or prices); detecting anomalies (from fraudulent banking transactions to red flags in a patient’s vital signs); determining what behaviors to adopt with customers (chatbots or virtual assistants) or work processes (advanced robotics). But how far has this potential for innovation already been tapped and turned into concrete business applications? What directions for AI development will we see in the future? And what criticalities?
When discussing the current state of AI technologies, the first thing to do is bust a myth: no AI system possesses the self-awareness or cognitive awareness that we humans do, nor is such an evolution likely to happen in the short- or medium-term, (Figure 2). This means that the human touch is still indispensible when developing AI applications for business. In other words, AI systems can reinforce and augment the cognitive capacity of analysts and managers – but cannot replace them, by any stretch of the imagination. This is particularly pertinent for upstream tasks, such as defining problems that we want AI to solve, but also in choosing, cleaning and categorizing data. Again, input from the people working on the project in question is essential. So AI projects are actually highly ‘human-intensive,’ and as such are potentially subject to human bias in terms of design and data input.
Figure 2 - The automation continuum
With this consideration as a starting point, the aim of the present research was to identify the key competencies that businesses need to support effective AI applications. By analyzing a number of concrete projects that are currently being developed, what emerges is that these competencies hinge on: statistics (the ability to work on models that process broad data sets), programming (working with AI languages), communication (indispensible for transferring inputs and knowledge to and from an extended set of in-house stakeholders), business (to fully understand how the company’s structures and needs), and design (establishing proper work flows). Based on these competencies, some areas of specialization can be identified: AI engineering; data science, data architecture, and problem modeling.
But today an obstacle to widespread dissemination of AI projects is the fact that specialists in these areas are hard to find. Added to this is the need to fully integrate AI experts into the organization to put them in the best position to communicate and interact with the rest of the management team and the work force, reengineering processes and redesigning activities if need be. So the real challenge of setting up AI projects in companies is mainly an operational and organizational one.
The second criticality has to do with the fact that AI technology is relatively immature as far as development techniques, infrastructure and applications. This means that the solutions that are currently being floated today are probably going to be outdated tomorrow (or the next day), making business investments in this field quite a risky proposition. That said, adopting shared, open-source solutions might be a way to mitigate the risk of premature obsolescence. And finally, proof positive of the relative immaturity of today’s AI solutions, most AI projects currently under construction are experimental or have a limited range of application, meaning they’re not (yet) scalable company-wide.
A third critical aspect ties into the ethical approach to building and using AI-based systems. The central question here is about responsibility, which brings up a series of decisions to disentangle, something that many companies are not yet ready to do. The classic debate revolves around who is responsible for an action taken by an ‘intelligent’ system, the human or the machine? This point is particularly relevant for areas of application like autonomous vehicles, where these ethical issues are literally ‘putting the brakes’ on mass adoption of self-driving cars.
Despite the limitations and uncertainties of current AI solutions, management should take the initiative right now and lay the groundwork for introducing AI in their companies. The impact of AI will be tremendous in terms of the dynamics, processes and opportunities in various business sectors. So the motto is ‘be prepared,’ and the key to doing so is active experimentation and capability building. This will also help companies build the baseline resources they need to capitalize on opportunities the moment they arise, as the technological evolution moves forward.
As far as future research, we need to: